
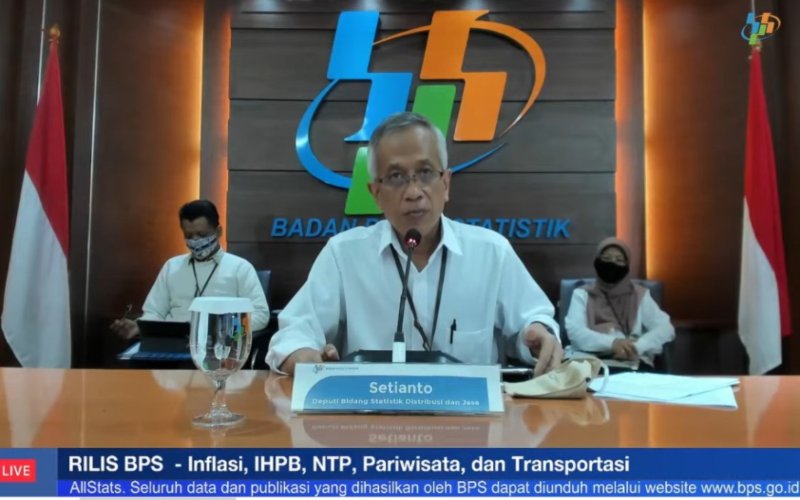
al-Uqud: Journal of Islamic Economics, 3(2), 174-187.įakhri, U. Comparison and Predicting Financial Performance of Islamic and Conventional Banks in Indonesia to Achieve Growth Sustainability. Accounting and Finance Research, 3(2), 124-128.įakhri, U.
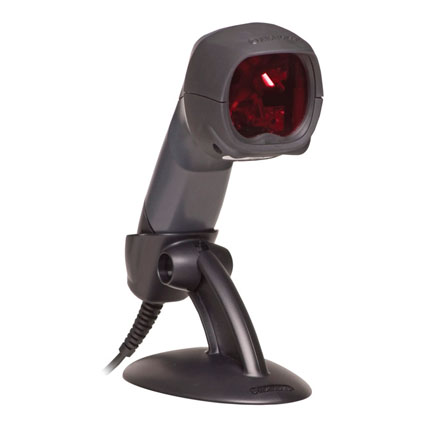
Bankruptcy prediction model using neural networks. Predicting bank financial failures using neural networks, support vector machines, and multivariate statistical methods: A comparative analysis in the sample of savings deposit insurance fund (SDIF) transferred banks in Turkey.

In 2010 International Conference on E-business, Management and Economics (IPEDR), 3.īank Indonesia. Performance comparison of multiple linear regression and artificial neural networks in predicting depositor return of Islamic Bank. Universitas Islam Indonesia, 7(2).Īnwar, S., & Watanabe, K. Analisis rasio keuangan untuk memprediksi kondisi financial distress perusahaan manufaktur yang terdaftar di bursa efek jakarta. In Proceedings of the 11th WSEAS international conference on Artificial Intelligence, Knowledge Engineering and Data Bases (AIKED'12), Cambridge, United Kingdom (pp. Evaluation of banks’ insolvency using artificial neural networks. ISRA International Journal of Islamic Finance, 12(3), 419-441Īl-Osaimy, M.H, (1998), Neural network system for predicting islamic bank performance, JKAU: Econ. The impact of economic blockade on the performance of Qatari Islamic and conventional banks: a period-and-group-wise comparison. This result is also in line with previous research where Islamic banking is more vulnerable than conventional banking in facing financial crises.Īli, A. Whereas in conventional banking, Operating Expenses to Operating Income was 72.87% and the Capital Adequacy Ratio was 17.31%. The results showed that the COVID-19 pandemic affected financial performance factors in the form of a Funding to Deposit Ratio of 35.21% Short Term Mismatch of 26.92% and Net Operation Margin of 26.92% in Islamic banking. Ratio (%), Short Term Mismatch (%) which are used as the independent variable, as well as Return on Assets which is used as the dependent variable. This study uses the Artificial Neural Network (ANN) method with 6 financial performance variables in the period of January 2020 - September 2020, namely Capital Adequacy Ratio (%), Operating Expenses / Operating Income (%), Net Operation Margin (%), Landing on Deposits. This study aims to determine the financial performance factors that are affected by the COVID-19 pandemic, both in Islamic and conventional banking which are included in the CBGB 2 category so that banks in Indonesia can anticipate it. The COVID-19 pandemic that is spreading in Indonesia has affected economic growth, likewise banks sector.
